Enhancing Efficiency with AI Search: Transform Your Information Retrieval Strategies
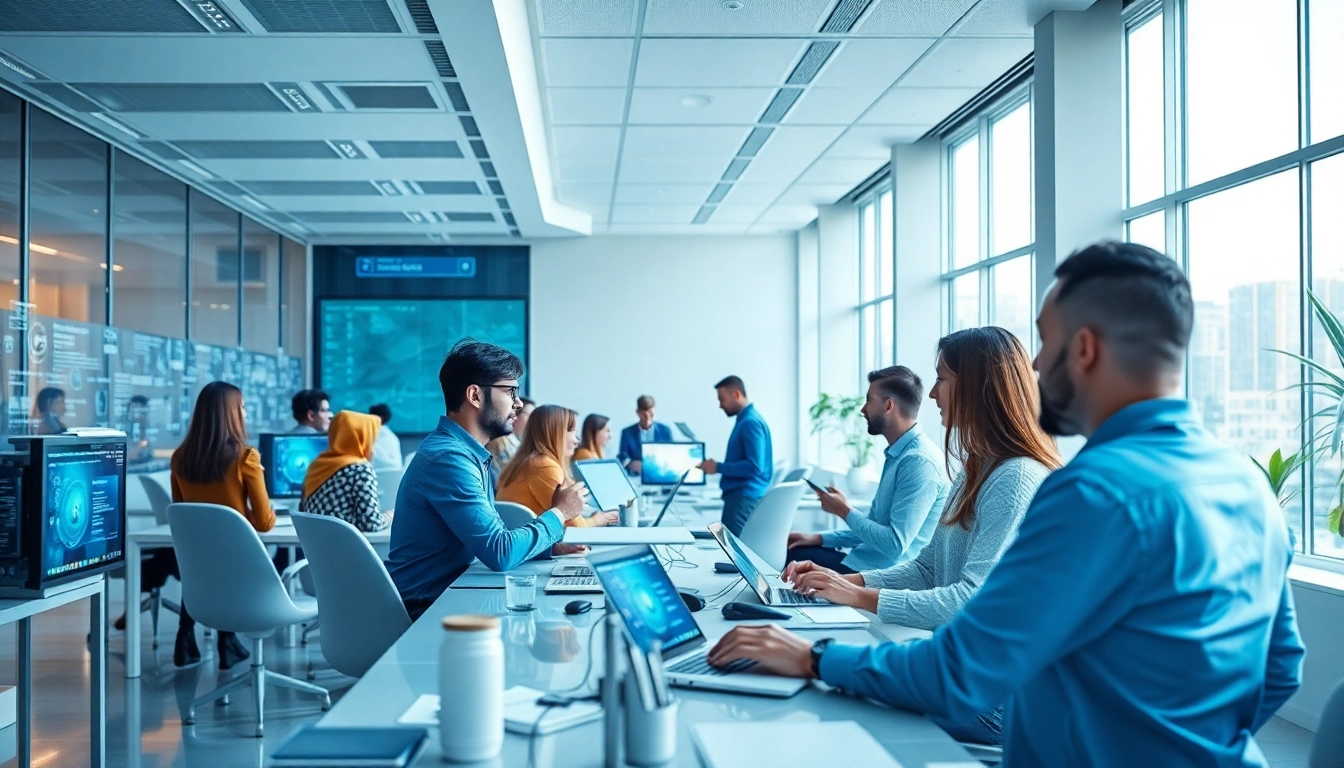
Understanding AI Search: What You Need to Know
Artificial intelligence (AI) has transformed many aspects of our everyday lives, from personal assistants to automated services, and is increasingly reshaping how we perform searches on the internet. The rise of AI search engines has resulted in a more nuanced approach to information retrieval, challenging traditional search mechanisms and offering enhanced capabilities. This article delves into the intricate landscape of AI search, exploring its foundational technology, distinguishing features compared to traditional search, and the multitude of benefits it brings to users.
The Basics of AI Search Technology
AI search technology employs machine learning algorithms and neural networks to improve the relevance and efficiency of search results. Unlike traditional search engines that typically rely on keyword matching and predetermined ranking algorithms, AI search engines analyze vast amounts of data to understand user intent and context better. This involves:
- Natural Language Processing (NLP): NLP allows computers to understand and interpret human language, enabling more conversational and contextual search queries.
- Machine Learning: Ongoing training on varied datasets helps AI systems learn from interactions and improve their accuracy over time.
- Semantic Search: This approach focuses on the meaning and context of the search query rather than just matching keywords, which leads to more relevant results.
Key Components of an AI Search Engine
An AI search engine is comprised of various interconnected components that work together to deliver high-quality search results. Key elements include:
- Indexing Systems: Efficient indexing systems organize vast repositories of information in a manner that allows for quick retrieval.
- User Interaction Layer: This layer interprets user input and queries, employing NLP techniques to understand user intent.
- Learning Algorithms: Algorithms that can learn from user feedback and improve continuous over time, adapting to changing user preferences and behavior.
- Personalization Engines: These customize results based on user profiles and previous interactions, making searches more relevant to individual users.
How AI Search Differs from Traditional Search
While traditional search engines primarily function through keyword matching and URL ranking systems, AI search represents a paradigm shift. The crucial differences lie in:
- User Intent Understanding: AI searches use contextual understanding to interpret what the user means rather than what they typed.
- Dynamic Result Generation: Results are continually updated and refined based on user interactions, unlike static ranking systems common in traditional searches.
- Multimodal Capabilities: AI search can handle various data types (text, images, voice), making it versatile in gathering accurate results from diverse inputs.
Benefits of Implementing AI Search Solutions
Speed and Accuracy in Information Retrieval
One of the most significant advantages of AI search solutions is their ability to retrieve information rapidly and accurately. By leveraging advanced algorithms, AI searches reduce the time it takes to find relevant data to mere seconds, which is an exponential improvement over traditional methods. The accuracy factor is equally crucial; AI search can sift through vast datasets and discern which results hold the most relevance based on user input and historical interaction data.
Enhancing User Experience through Personalization
The user experience is fundamentally enhanced through personalization features integrated into AI search solutions. These customized experiences ensure that users receive content tailored to their interests and past behaviors. For instance, a user frequently searching for technology trends will see more relevant articles related to tech compared to someone whose interests lie in cooking or wellness. This tailored approach not only improves satisfaction but also promotes user engagement.
Reducing Overload with Advanced Filtering Capabilities
As information overload becomes a common issue, AI search engines come equipped with advanced filtering capabilities designed to refine search results. Users can apply various filters to hone in on precisely what they are seeking, be it by content type, publication date, or relevancy score. This filtering is particularly beneficial in professional and academic settings, where precision is paramount for efficient decision-making.
Best Practices for Using AI Search Effectively
Optimizing Queries for AI Search Engines
To achieve the best results from AI search engines, users must optimize their queries effectively. This entails using complete sentences and questions, as opposed to fragmented phrases. For example, instead of typing “AI search tools,” the user might ask, “What are the best AI search tools available in 2025?” This change in query structure helps the AI better understand the context and provide relevant answers.
Utilizing Natural Language Processing Features
Taking advantage of natural language processing features can significantly enhance search results. Users should interact conversationally with AI search engines, utilizing contextual cues and elaborating on their inquiries for improved accuracy. This allows the AI to recognize nuances in language and derive more relevant information tailored to user needs.
Common Mistakes to Avoid in AI Search Implementation
When integrating AI search solutions, several common pitfalls can hinder effectiveness. These include:
- Neglecting Data Quality: Poor data quality can lead to inaccurate results and erode user trust. Invest in robust data-cleaning processes.
- Ignoring User Training: Users must understand how to leverage AI search tools to their full potential. Training is vital for adoption and efficiency.
- Overlooking Privacy Concerns: Ensure compliance with data privacy regulations and consider user privacy will promote trust in AI applications.
Case Studies: Successful AI Search Implementations
Enterprise Solutions and Their Impact on Productivity
Several enterprises have successfully implemented AI search solutions, significantly enhancing productivity across departments. For instance, a global consulting firm integrated AI search into its knowledge management system. By allowing employees to query millions of documents and case studies more intuitively, the firm reduced the time spent searching for information by over 40%, enabling consultants to focus on strategy and delivery rather than data retrieval.
Academic Institutions Leveraging AI Search
Universities and research institutions are harnessing AI search technologies to provide students and faculty with advanced research tools. A notable example is an AI-enhanced library search system deployed by a leading university, allowing scholars to query resources in a more human-centric way, gaining quick access to online journals and articles relevant to their studies. This initiative has improved research efficiency and has encouraged interdisciplinary studies by exposing users to diverse materials.
Real-World Applications in E-Commerce and Marketing
E-commerce platforms are employing AI search to deliver personalized shopping experiences. By understanding user intent and purchase behavior, companies like Amazon can recommend products dynamically. A case study in the fashion retail sector showcased how AI-powered search algorithms enabled users to find items based on images they uploaded, drastically increasing conversion rates and customer satisfaction. Marketers, in turn, have used these insights to target ads more effectively, aligning product recommendations with customer needs.
Future of AI Search: Trends and Innovations
Emerging Technologies in AI Search
The future of AI search is bright and filled with innovative technologies that will redefine how we access and interact with information. Key emerging trends include:
- Multimodal Searches: The integration of text, voice, and visual search capabilities is evolving, allowing users to search through images and voice commands.
- AI Ethics and Accountability: As AI technologies evolve, ethical considerations regarding biased data and decision-making transparency are becoming paramount, necessitating comprehensive frameworks.
- Augmented Reality (AR) Integration: AI search engines may soon incorporate AR elements to visualize information in interactive environments, enhancing user engagement.
Predictions for the Next Five Years
Looking ahead, we anticipate a seismic shift in AI search applications. By 2028, it’s projected that AI search engines will dominate the online search landscape, where traditional search engines may adapt or risk obsolescence. Enhanced personalization, adaptive learning from user behaviors, and improved natural language interfaces will define this new frontier. Furthermore, the convergence of AI with blockchain technology may facilitate better data integrity and trust in search results, creating a safe environment for users.
The Role of AI Ethics in Search Evolution
AI ethics will take center stage in the development of AI search technologies. With increasing awareness around data privacy and algorithmic bias, companies will need to prioritize ethical guidelines in their AI implementations. These guidelines will focus on ensuring that AI search respects user privacy rights, maintains transparency in data usage, and mitigates biases in algorithmic outputs. Ultimately, investing in a responsible AI foundation will not only protect users but also foster trust and credibility in emerging AI search solutions.
Leave a Comment